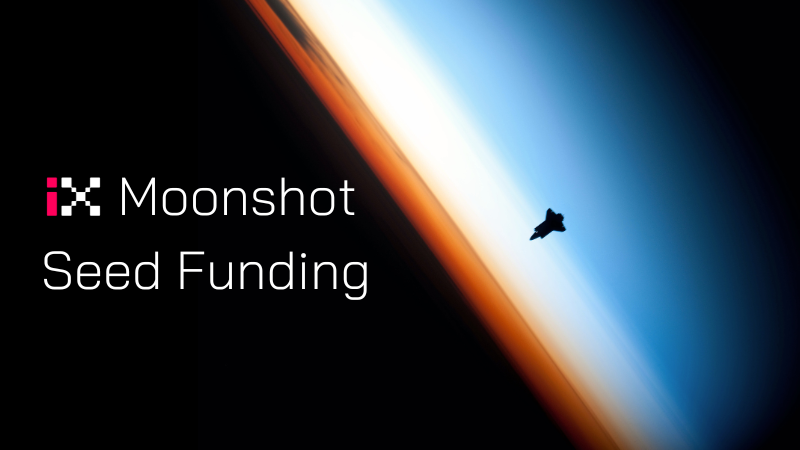
We are delighted to announce that I-X will support seven research projects in the second round of its Moonshot Seed Funding scheme, co-sponsored by I-X and the I-X Centre for AI in Science. Thanks to the generous contribution from the I-X Centre for AI in Science, in this round we were able to fund even more outstanding projects led by Early Career Researchers from our community.
The initiative is a part of I-X’s commitment to delivering ambitious scientific “moonshots”, by bringing together multi-disciplinary academic teams to tackle problems that they cannot solve alone. The scheme provides small amounts of seed funding to help the I-X community initiate new collaborations in order to achieve these “moonshots”.
The Moonshot Seed Funding supports various projects, including creating proof-of-concept prototypes, hosting grant writing workshops, running small field experiments, hiring interns, or purchasing research equipment for the I-X labs. Each project is led by a member of the I-X community; to further I-X’s commitment to supporting upcoming researchers’ development, each project aims to involve an Early Career Researcher—PhD student, postdoctoral researcher, or junior member of the faculty—as a co-lead.
The last round of winning projects included work on non-invasive multi-modal prognosis of traumatic brain injury, autonomous underwater inspection platform, and prototyping acoustic sensors for scalable acoustic monitoring of species.
Winning Projects
We are excited to introduce the projects that have been awarded funding in this round:
-
C.A.L.C. Project
This project, led by Dr Tao Bi, aims to explore the role of conversational agents in supporting caregivers to accurately capture, report, and communicate health symptoms for dependents with limited or no self-reporting and communication ability. The case study will be based on pet caregivers.
-
Building a Physical Platform for Testing Online Learning and Reinforcement Learning Algorithms
This project, led by Dr Lloyd Fung, aims to build a physical cart-pole system with several arms to test virtually trained machine-learning algorithms in the real world.
-
Evolving Neural Networks through Augmenting Modules
This project, led by Dr Marcus Ghosh, aims to develop new algorithms for evolving neural network architectures. Exploring these architectures will generate insights into how network structure relates to function and may catalyse breakthroughs in machine learning.
-
Bayesian Optimisation for the Discovery of Supramolecular Assemblies
This project, led by Dr Austin Mroz and Annabel Basford (based in the Greenaway and Jelfs groups in Chemistry), aims to establish and demonstrate the first hybrid automated workflow capable of accessing and optimising the synthesis of promising discrete supramolecular assemblies for target applications. This will be achieved by integrating bespoke optimisation algorithms with automated high-throughput experimental screening and data analysis.
-
Toward Generalist Hallucination Detection in Large Language Models for Reliable Deployment in High-Stakes Domains
This project, led by Mengjia Niu, aims to develop a generalizable approach that is capable of detecting previously unseen hallucinations in large language models, maintaining effectiveness across different application domains.
-
Beyond Loss: A Graph Theoretic Approach to the Learning Dynamics of LLMs
This project, led by Dr Sara Veneziale, aims to discover how recording and analysing graph features during LLM training can be used to detect promising hyperparameters early, providing machine learning practitioners with new tools that can lead to cost savings and more effectively trained models.
-
Autonomous Pipe Inspection Microbots
This project, led by Houzhe Wang, aims to explore AI and autonomous robotic (topological SLAM) technologies for enabling a robot to locate itself in a confined pipe network containing water and map the complex connectivity.
Huge congratulations to all the winners!
If you are interested in joining the I-X Community, consider applying for the I-X Affiliate Scheme, which is open to Imperial College London research staff.