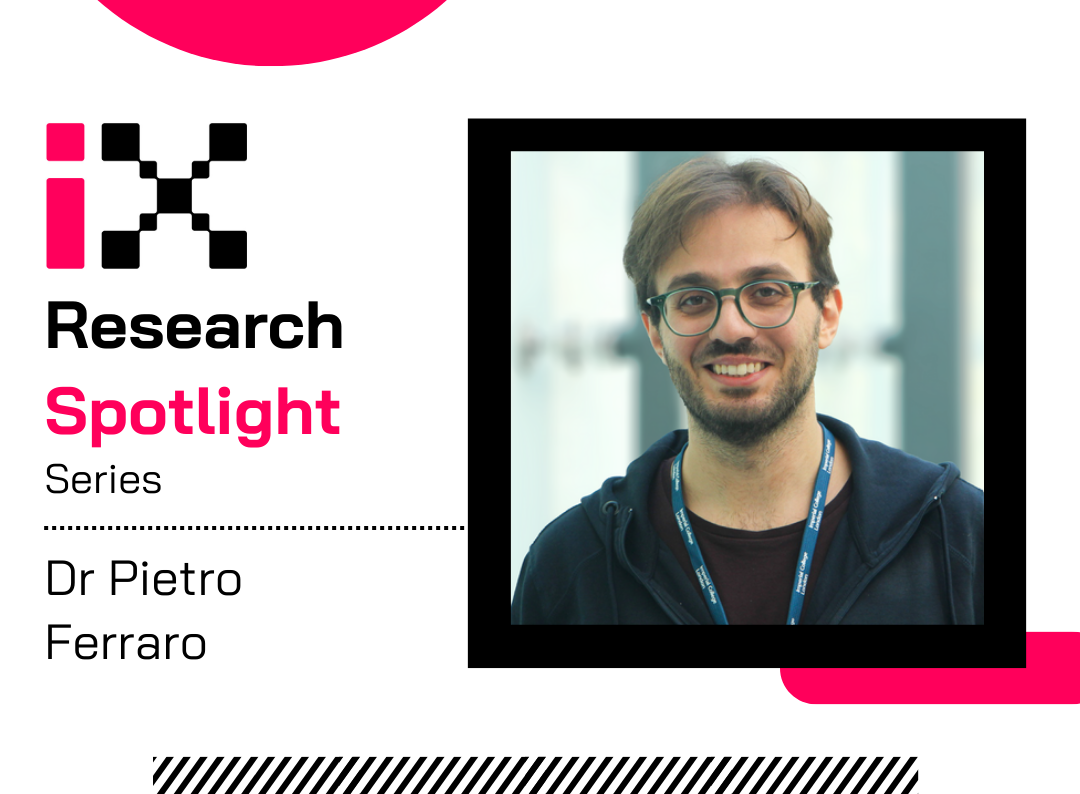
We are excited to introduce a new series on our website, I-X Research Spotlight, which explores cutting-edge AI and ML research conducted by I-X faculty members. Kicking off the series is an insight into the research of Dr Pietro Ferraro.
Pietro joined Imperial as a Research Fellow in 2019, after spending a year as a postdoc at the University College Dublin. Before that, he completed PhD in Electrical and Control Engineering at the University of Pisa. In 2023, he was promoted to a Lecturer in Artificial Intelligence and Machine Learning at I-X and Dyson School of Design Engineering. His research interests include reinforcement learning and control theory, particularly its applications to the sharing economy domain.
The Art of Optimisation
Have you ever wondered how to determine the optimal drug dosage for a patient based on their health condition? Or how to choose the best charging solution to provide maximum cost-efficiency for a fleet of electric vehicles? Or how to programme traffic lights to ensure the lowest possible CO2 emissions? All these questions are essentially optimisation problems. And while finding a solution to these complex issues is difficult, the real challenge lies in finding solutions that are most effective. One way to tackle this challenge is by utilising machine learning and artificial intelligence. But how can we ensure that these technologies provide not only optimal solutions but also safe ones? Dr Pietro Ferraro’s research aims to answer this question.
Safe Reinforcement Learning
For Pietro, research is about problem-solving. Reinforcement Learning (RL), which he specialises in, is one of the means to solve complex problems. RL is a machine learning method designed to train software to make optimal decisions. However, while RL proves to be a powerful way to control dynamic systems, there are certain risks associated with it. Due to its learning mechanism emulating the trial-and-error learning process used by people, RL may lead to unpredictable actions, which can compromise the safety of critical systems. “A self-driving car is a great way to picture how RL operates,” Pietro explains, “the car learns through trial and error, figuring out how to steer, accelerate, and brake to avoid obstacles while reaching its destination. Over time, it discovers the best actions to maximise safety and efficiency without being explicitly programmed for every situation. While this sounds great in theory, the cost for it is that the car will commit a huge amount of mistakes, some of which might have disastrous consequences, before learning appropriate actions.”
In his research, Pietro explores ways to make RL agents learn to optimally solve tasks without jeopardizing the system’s safety. One such approach is RL with Adaptive Control Regularization (RL-ACR), an algorithm that ensures safe RL exploration by integrating the RL policy with a policy regulator, which enforces hard-coded safety constraints. In a recent paper, Pietro and his research group showed that RL-ACR not only guarantees safety during training but also matches the performance of other RL approaches that overlook safety. By introducing two parallel agents—a safety regulator and an adaptive agent—the model is capable of finding optimal solutions without implementing policies that may harm the system or the agent itself. Reflecting on the impact of safe RL solutions, Pietro said: “Today, despite its potential, RL remains not applicable to a large number of domains where the safety of the equipment or of the people interacting with it are at stake. It is therefore extremely important to develop techniques that ensure that these algorithms operate within the safety boundaries imposed by their designers.”
From Theory to Practice
While Pietro is deeply interested in the theoretical foundations of reinforcement learning, for him theory is only an entry point. Rather than solving theoretical problems, his research explores how theory can inform the development of real-life solutions to some of the biggest societal and industrial challenges.
Pietro works on CoDiet, an international research project aiming to address diet-related diseases, including diabetes, obesity, and heart conditions. This interdisciplinary project utilises diet-monitoring technologies first to understand the link between our diet and certain illnesses, and then, using RL, develop AI tools that craft tailored dietary plans for individuals based on their health profile. The ultimate aim is to enhance people’s health through personalised nutrition. Reflecting on the potential of AI and ML in enhancing society’s health and bringing wider societal benefits, Pietro said: “According to the World Health Organisation, non-communicable diseases kill 41 million people each year, equivalent to 74% of all deaths globally. Codiet aims to apply data-driven techniques and AI methods to enhance our understanding of these diseases and tailor personalised interventions to prevent them.”
Problem-Solving
Having a background in engineering, Pietro has always been interested in solving industrial problems. One such challenge was how to make electric vehicles charging quicker and cheaper. To tackle this, Pietro together with Professor Robert Shorten, Andrew Cullen, Hugh Sheehy and John Goodbody, created Go Eve. The start-up produces DockChain, a patented rapid charging system for electric vehicles, that enables quick charging of not two but multiple cars simultaneously. DockChain is essentially “a charge point multiplier”. In other words, it enables multiple parking bays to have access to electricity by using a daisy chain of charging points that are connected to a single base power source. While the charge points look similar to standard slow AC charging points, they have access to a high-power DC charger, providing higher flexibility and lower operational costs. Additionally, since every parking spot can be used to charge electric vehicles, the technology also solves logistical issues, such as finding a place to charge a car and having to move vehicles after they are done charging.
When Pietro and the team started Go Eve in 2021, their goal was to create a robust technology able to solve the problem of charging spots availability and reliability. However, this was only a starting point. Another problem that the team aims to solve is the economic one: ensuring that cheap and green energy is used to charge cars. The company is now working on further upgrades to its product, exploring the potential to use AI to maximise customers’ revenue by charging their fleet at optimal times and selling unused energy back to the grid. Thinking about the impact of AI on DockChain technology, Pietro said: “AI and RL provide an exceptional framework to solve complex scheduling problems such as the ones that our clients are facing when presented with the challenge of managing a fleet of EVs, renewable energy sources and their intricate interaction.”
AI & Real-Life Solutions
In his work, Pietro also wants to empower students and young academics to think about research as a way to bring real-life solutions. One of his recent MSc students completed a thesis on using ML to minimise the impact of non-exhaust emissions in cities by controlling traffic lights.
Looking ahead, Pietro hopes to solve some of the main blockers that prevent the application of AI solutions to many real-life problems: “While AI has the potential to revolutionize society as we know it, we still have a large number of issues that require practical and robust solutions. Safety, interpretability, and alignment are not nice-to-have properties but fundamental requirements of real-world AI solutions.”