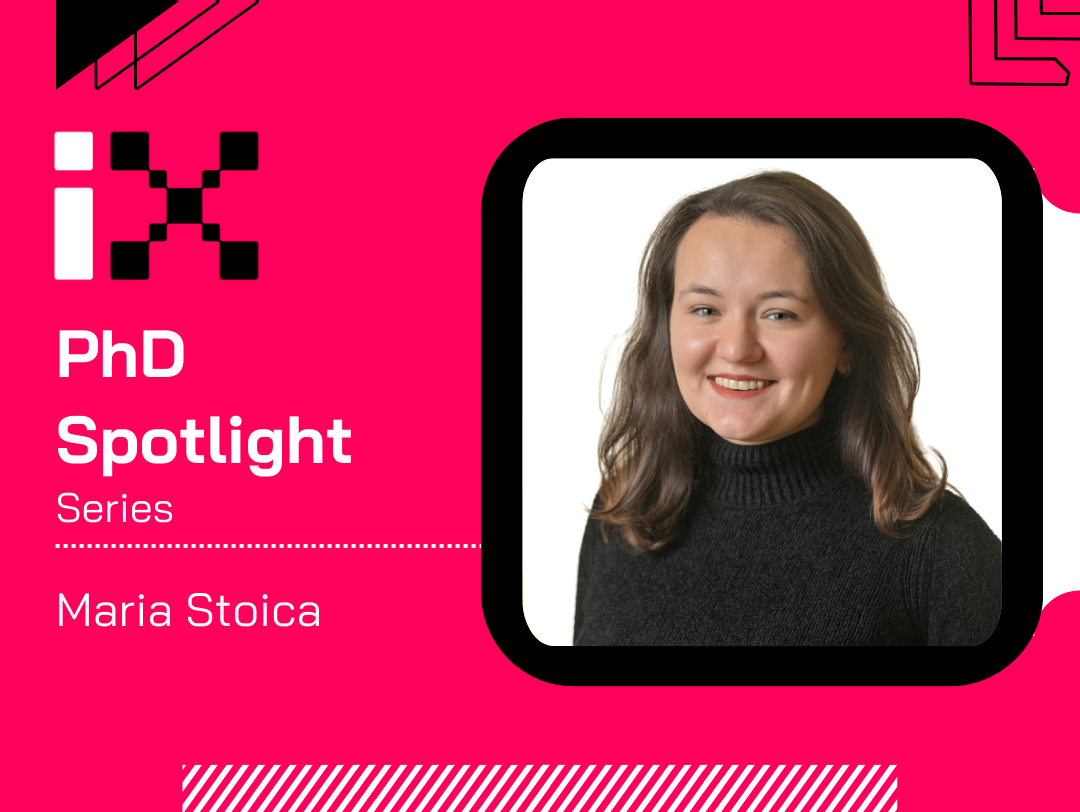
In the I-X PhD Spotlight series, we ask our PhD students about As & Is of their research.
In today’s interview, we feature Maria Stoica, a PhD student at I-X and the Department of Computing. Maria works on the monitoring of AI systems in the Safe Artificial Intelligence Lab (SAIL) led by Professor Alessio Lomuscio. She is also part of the Centre for Doctoral Training in Safe and Trusted AI. Before joining Imperial, she completed a bachelor’s degree in computer science at Harvard and then joined the financial services industry for four years working in roles in commodities and foreign exchange markets. She then completed a master’s degree in advanced computer science at Oxford University.
AI #1: Application & Innovation
Could you tell us a bit about your PhD project and its practical applications?
My PhD research addresses a critical challenge in artificial intelligence: ensuring the reliability and safety of neural networks when deployed in real-world scenarios. I am developing lightweight and accurate monitoring algorithms to detect out-of-distribution inputs and unexpected behaviours in neural networks. These situations, where inputs deviate significantly from the data seen during training or the model behaves unpredictably, can lead to failures with potentially severe consequences in high-stakes applications. My goal is to create tools that operate efficiently in real-time, running alongside the neural networks without adding significant computational overhead, to enhance their safety and robustness.
The practical applications of my work span a wide range of industries where safety and reliability are critical. In autonomous driving, for example, monitoring algorithms could identify unusual road conditions or objects, triggering fail-safes before critical errors occur. In healthcare, they could flag anomalies in medical image analysis systems, ensuring that rare conditions or unexpected data distributions are brought to the attention of medical professionals. Beyond safety-critical domains, my research has the potential to enhance trust in AI systems by providing transparency and early warnings for unexpected model behaviour in areas like finance and cybersecurity. I aim to make machine learning a more dependable tool by enabling safer and more reliable AI systems.
How does your project contribute to driving innovation in your field?
Creating lightweight, real-time monitoring algorithms that can operate alongside neural networks without imposing significant computational or operational overhead is the main challenge and innovation of this work. Unlike other approaches, such as verification, that are often resource-intensive or retroactively analyse model behaviour, my work integrates monitoring directly into the deployment pipeline to provide immediate feedback on out-of-distribution inputs or unexpected behaviour. This real-time capability ensures safety and robustness in safety-critical environments like autonomous vehicles or healthcare systems. Additionally, my project combines techniques from multiple disciplines, including statistical analysis, anomaly detection, and explainability in AI, to build efficient and interpretable solutions.
AI #2: Area & Impact
What motivated you to work in this area?
During my experience in the finance industry, I witnessed firsthand how critical trustworthiness is in real-world machine learning applications. In finance, decision-making systems must operate reliably under high stakes, as errors or unexpected behaviours can lead to significant financial losses or undermine client confidence. This exposure highlighted the importance of creating systems that are not only effective but also robust and transparent. Seeing these challenges in action inspired me to pursue research focused on ensuring the safety and reliability of AI systems, especially as they are increasingly deployed in complex and high-impact environments.
How do you think your research could impact industry and society?
My research aims to redefine how organisations deploy and maintain AI systems by offering robust safety mechanisms and explainability tools that integrate seamlessly with existing models. Industries such as finance, healthcare, transportation, and cybersecurity could benefit from these advancements by reducing risks associated with system failures or unexpected behaviours. Moreover, including explainable monitoring ensures end-users and stakeholders gain more precise insights into AI decision-making processes, promoting transparency and accountability. By enabling safer and more interpretable AI systems, my research aims to bridge the gap between technological advancement and societal acceptance, paving the way for a future where AI can be trusted to operate responsibly in critical and sensitive environments.
AI #3: Advice &
I-X
What advice would you give to someone considering a PhD in your field?
It is important to have a deep curiosity for AI’s theoretical and practical aspects. This field often requires a strong understanding of machine learning principles, statistical analysis, and programming and the ability to think critically about real-world challenges. Also, it helps to be prepared to embrace setbacks as part of the process. Research in this field is iterative, and learning from failures is a significant part of making meaningful progress. Stay focused on the long-term impact of your work, as the opportunity to contribute to shaping the future of AI makes this path incredibly rewarding.
What is the most fun about being part of the I-X?
It is really nice being a part of an interdisciplinary research community and seeing how different groups and departments view the challenges of AI. There are a lot of events and socials that help foster this community, and there are many ways to get involved! I recently was able to speak to a group of A-level students about my research and it was a wonderful opportunity to see how excited the next generation is about AI!