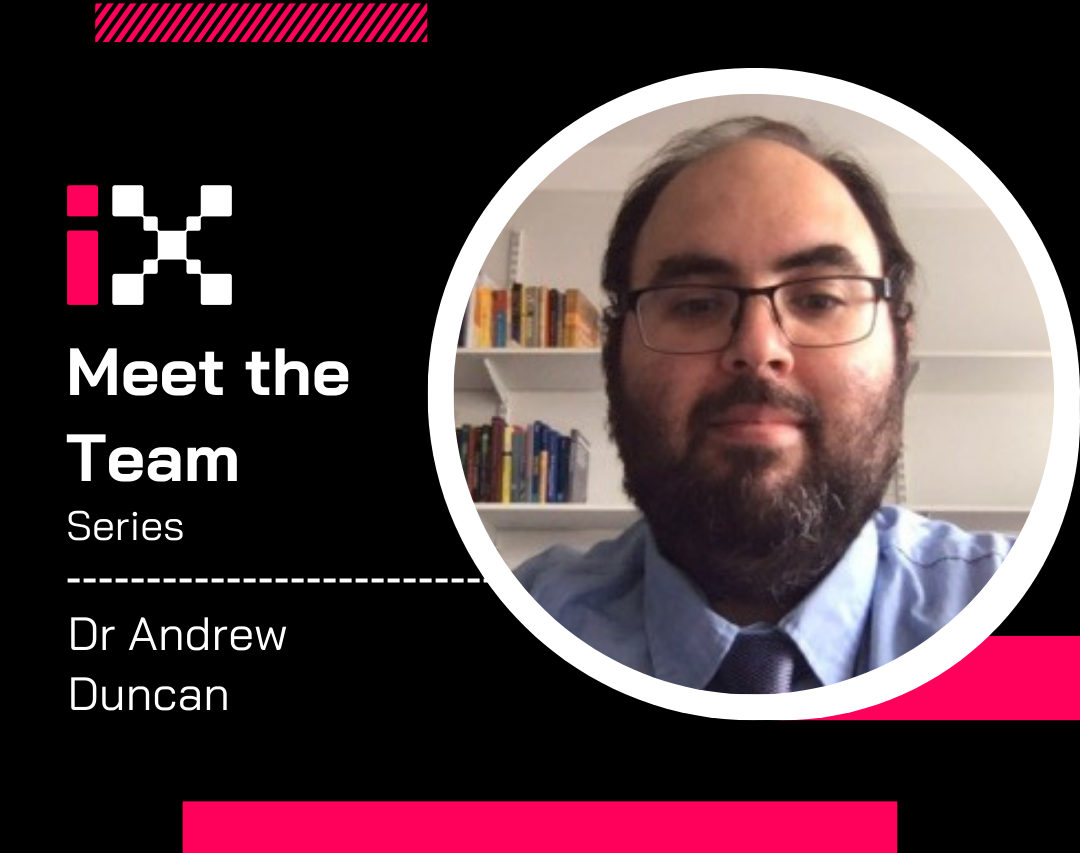
We are excited to welcome Dr Andrew Duncan to the I-X Team! Andrew is a Senior Lecturer in Statistics and Data-centric Engineering at I-X and the Department of Mathematics.
* * *
Welcome to the I-X team! Could you tell us a bit about yourself and your academic background?
Thanks! My background sits at the intersection of Computational Statistics and Applied Mathematics. I started with a joint degree in Mathematics and Computing in Malta, then moved to the UK, where I completed a PhD in Mathematics in 2014. My doctoral research focused on stochastic partial differential equations, with an applied emphasis on reducing the computational cost of large-scale stochastic simulations in cellular biology.
Following my PhD, I held postdoctoral positions at Oxford and Imperial, gradually shifting my focus toward statistical machine learning. In 2016, I joined the University of Sussex as a Lecturer in Statistics and Probability, and in 2018, I moved to the Statistics Section within the Mathematics Department at Imperial.
In 2021, I took a two-year break from academia to work in the tech sector, applying simulation, computational statistics, and AI to complex challenges in defence and national security.
In 2024, I became Director of Fundamental Research at the Alan Turing Institute, helping lead its strategic transformation. In that role, I restructured the division to create dedicated teams focused on AI for Physical Systems, Decision-Making and Game Theory, and Large Language Models—with a strong emphasis on democratising AI through open-source tools and model development.
I’m now returning to Imperial full-time, and I’m delighted to be joining the I-X team!
What projects are you working on currently and what part of your work is most exciting for you right now?
Much of my current research is driven by a fascination with probabilistic generative models (PGMs)—their underlying theory, practical methodologies, and broad range of applications. I’m especially interested in how PGMs can power downstream tasks such as optimization, risk-informed decision-making, and scientific discovery. What excites me most is their potential to address complex challenges at the intersection of data and the physical laws governing scientific and engineering systems.
In many scientific and engineering domains, numerical simulations have long served as a powerful lens into the structure and dynamics of intricate physical systems. When enriched with data, these simulations become a kind of “crystal ball”, offering insight into future behaviour. Yet, the sheer computational cost of large-scale simulations remains a major hurdle—particularly for systems that are inherently multi-scale or governed by multiple physical processes.
My research is driven by the question: how can we harness machine learning—especially PGMs—to augment, accelerate and improve these simulations? By integrating data, simulation, and ML, how can we build tools that unlock new scientific insights, while also lowering the barrier for labs with limited computational resources to participate in cutting-edge research.
A significant part of my work is collaborative, involving partnerships with other research groups and organisations. For instance, I’m currently one of the investigators in an MSCA-funded consortium led by Toyota Motor Europe, where I’m exploring the use of generative models to accelerate the design workflow for Proton Exchange Membrane Fuel Cells (PEMFC). More recently, I’ve also been developing collaborations with the UK Atomic Energy Authority, investigating how AI and machine learning can support and accelerate design optimisation efforts.
In your research, you work on applications of artificial intelligence across various field: from cellular biology, through chemical engineering, to aerospace and energy. How do you envision the role of AI and ML evolving in industrial applications over the next few years? Are there any emerging trends or technologies that you find particularly promising?
There has long been a tradition of applying artificial intelligence and machine learning to challenges in the natural sciences and engineering—with varying levels of success over the years. But recent advances in computational hardware, the availability of large-scale datasets, and the explosion of high-quality open-source tools have dramatically raised the level of ambition in this space.
The emergence of large-scale models like AlphaFold 3, Evo 2, and MatterGen has shown that, with sufficient data and physical guidance, the fundamental structure and dynamics of the physical world can be learned by these models —far more effectively than many of us had previously imagined. These breakthroughs are shifting the baseline for what’s possible in scientific discovery and design.
Now, many research communities and organizations are asking a new question: “We’ve built a foundation model—what comes next?” Probabilistic generative models are not, by themselves, decision-makers. To be useful in practice, they must be embedded within broader scientific workflows—helping to generate hypotheses, design and interpret experiments, and iteratively refine our understanding of complex systems.
Fortunately, many of the underlying methods and principles are shared across domains, making a multidisciplinary approach essential. Yet despite this, I’ve often seen AI/ML efforts held back by disciplinary silos—where different fields independently reinvent solutions rather than leveraging parallel developments elsewhere. One of the key barriers is a lack of appropriate venues to share and disseminate AI for Science results across disciplines.
This is precisely what motivated me to take on the role of Editor-in-Chief of ACM’s new journal Transactions on AI in Science, together with Nick Jones (Imperial College London) and Siva Rajamanickam (Sandia National Laboratories). Our goal is to create a dedicated platform for rigorous, cross-cutting research at the intersection of AI and science.
What attracted you to working at I-X?
I’m a firm believer in mission-driven research that spans the full journey from whiteboard to real-world deployment. My work moves across this spectrum—from developing fundamental mathematical and statistical principles to tackling practical challenges in applied scientific and engineering contexts. What draws me to I-X is its deeply multidisciplinary culture and its commitment to both the theoretical foundations and impactful applications of AI, ML, and Statistics—an approach that strongly aligns with how I think and work as a scientist.
What are your interests outside your work?
Most of my free time is spent with my family, which typically means family outings or playing Minecraft with my son. Other than that, I love to build things, and I usually have multiple side projects exploring fun little tech projects, from software to hardware.